Using AI to Optimize Resources
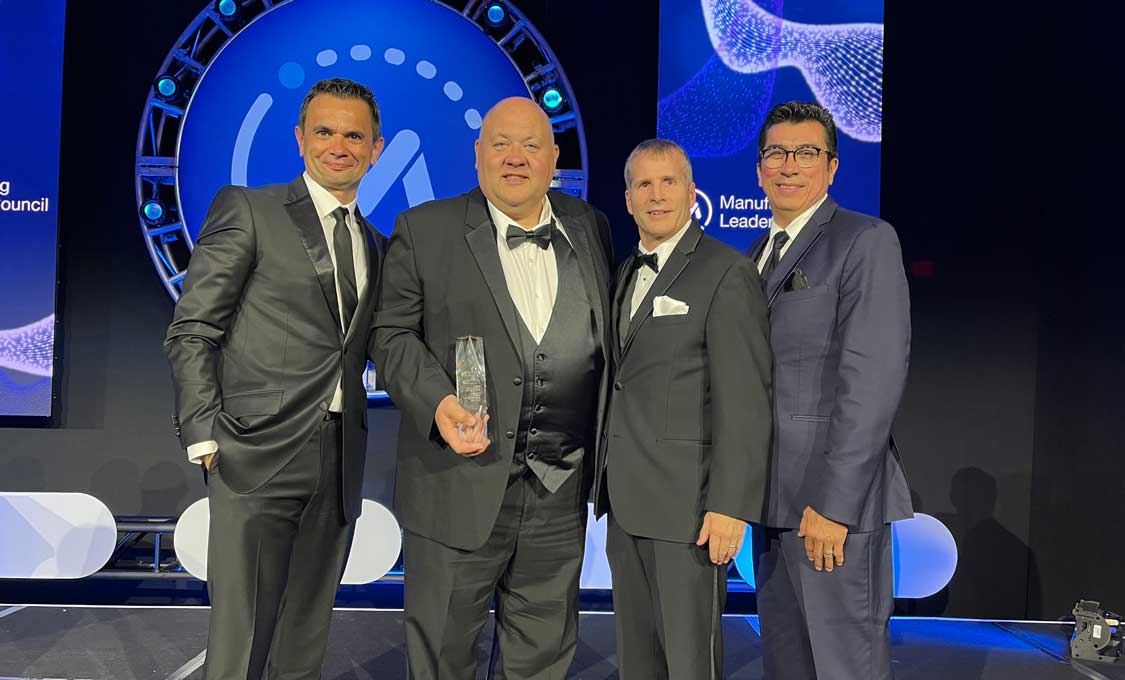
Lead oxide is the single largest material component required for battery manufacturing and demand for the metal is outpacing supply.
At Clarios, we meet this demand in two ways: producing our own oxide using our oxide mill equipment in our facilities and by purchasing it from third-party vendors. While making our own oxide is the most cost-effective choice, our production couldn’t keep pace with increasing demand, forcing us to rely on outsourced material. Normally, the solution to this would be to increase our capacity by installing more mills, but instead our team committed themselves to finding a sustainable solution using the power of generative machine learning, also known as artificial intelligence (AI).
Our Operations and IT teams partnered to use automated learning to comb through billions of data points from our oxide mills at our St. Joseph. Missouri and Winston-Salem, North Carolina facilities. Using this advanced analytics tool, the team uncovered previously inaccurate feedback data used by plant operators and existing machine controls to optimize throughput.
“It was like magic to me,” said Steve Turner, Director of Process Excellence. “The new AI and machine learning capabilities that our IT partners brought to the team opened a new world of analytics we couldn’t access before. In the past, we might have had 50 input variables, each with hundreds of thousands of data points and we’d use our intuition to pick three to five to analyze, which would take weeks. Now we’re able to process all of it. We wouldn’t have come to the conclusions we did without it.”
After validating the new controls with more reliable feedback on one mill, the approach was deployed to other mills, improving process stability. The project broke throughput records and achieved an annual savings rate of more than $3.1M by reducing our reliance on third party lead oxide.
The changes implemented by the team made the decades-old equipment more efficient than when it was originally installed 20-25 years ago. In addition to rolling out the process to other plants, these changes have also revealed opportunities to unlock design performance for more efficient and sustainable use of materials.
This project supported our sustainability goals in multiple ways: making our process more efficient to stretch our raw materials further and improving our process rather than needlessly replacing equipment.
For their efforts, the team was recognized by the Manufacturing Leadership Council as a Finalist in the AI and Machine Learning category of the 2023 Manufacturing Leadership Awards.
Learn more about our innovative environmental stewardship work in our 2023 Sustainability Report.